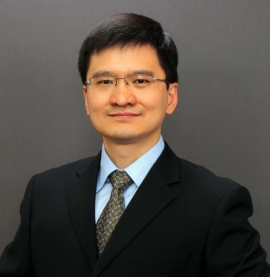
Dr. Long’s research purposefully includes novel statistical and machine learning research and impactful biomedical research, each of which reinforces the other. Its thrust is to develop statistical and machine learning methods and tools for advancing precision medicine and population health. Specifically, he has developed methods for analysis of big health data including, but not limited to, -omics data, electronic health records (EHRs) data, and mobile health (mHealth) data, missing data, causal inference, data privacy, data and algorithmic fairness, Bayesian methods and clinical trials. Dr. Long’s methodological research has been supported by the National Institutes of Health (NIH), the Patient-Centered Outcomes Research Institute (PCORI), and the National Science Foundation (NSF).
Dr. Long has directed the Statistical and Data Coordinating Center for national research networks and large-scale multi-site clinical studies—supervising a team of database administrators and programmers, application developers and statistical analysts. He currently co-directs (with Dr. Nicola Mason at Penn Vet) the Coordinating Center for the Premedical Cancer Immunotherapy Network for Canine Trials (PRECINCT), part of NCI’s Cancer Moonshot Initiative.
Dr. Long is the founding Director of the Center for Cancer Data Science, and Associate Director for Cancer Informatics of the Penn Institute for Biomedical Informatics. He also directs the Biostatistics and Bioinformatics Core in the Abramson Cancer Center at the University of Pennsylvania.
Dr. Long is an elected fellow of the American Association for the Advancement of Science, elected fellow of the American Statistical Association and elected member of the International Statistical Institute.
Read more about Dr. Long's research.
Content Area Specialties
cancer; cardiovascular diseases; neurological disorders and neurodegeneration; diabetes; kidney diseases; mental health
Methods Specialties
Statistical and machine learning methods for analysis of big health data (such as -omics, electronic health records, and mobile health data); missing data; causal inference; data privacy and algorithmic fairness; Bayesian methods; clinical trials